Blog post
What Birthdays Do Synthetic Fraudsters Choose?
Charlie Custer
Published
October 10, 2024
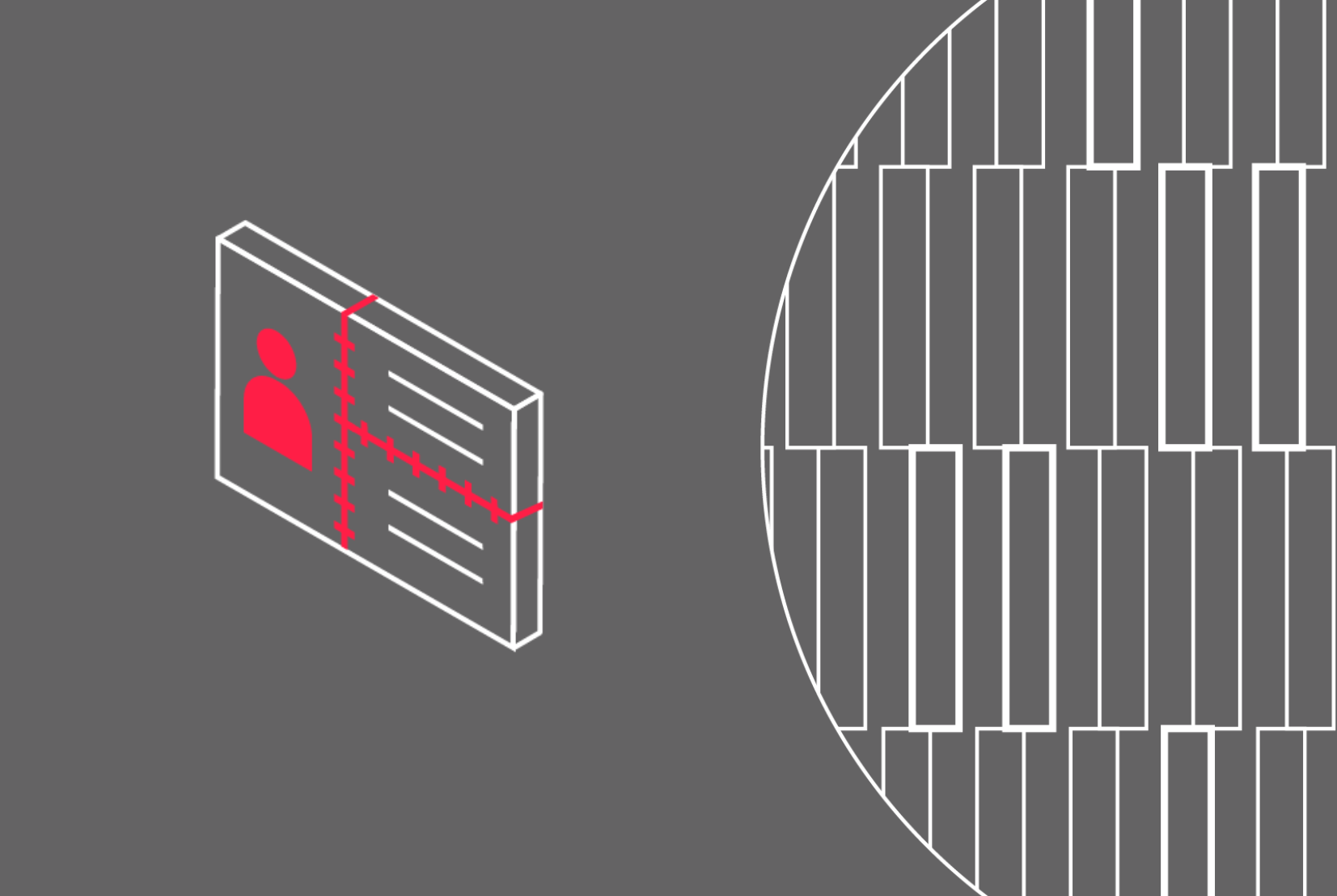
Third-party synthetic fraudsters invent identities out of whole cloth, making up names, SSNs, and dates of birth to create fake identities they can use to secure credit and (ultimately) potentially defraud a wide variety of institutions.
It's a serious problem, and one that we spend a lot of time thinking about. At SentiLink, our focus is catching synthetic fraudsters at the point of application, but in our downtime it's also fun to investigate the identities they come up with. For example: since they can choose any DOB they want, what "birthdays" do they choose, and why?
A quick disclaimer
This is just an analysis we did out of curiosity. Specific birthdays are not a part of SentiLink's fraud detection models or any of our fraud prevention products. We would not recommend that anyone looking to catch synthetic identities base their analysis on birthdays.
Methodology: investigating synthetic DOBs
To answer the question of whether synthetic DOBs differ from real-world DOBs, we looked at a sample of two million applications from SentiLink's data warehouse:
- 1M applications our models indicate are likely Third-Party Synthetic.
- 1M applications our models indicate are likely clear (real people).
After filtering to remove duplicates and ensure that each unique identity was counted only once (some identities had multiple applications), we were left with a dataset of 722,470 synthetic identities and 582,030 real identities.
For context, SentiLink works with a variety of partners, but many are banks, credit unions, and other financial institutions, so this data comes primarily from the population of credit-active Americans.
The most and least common real birthdays
To understand how synthetic birthdays might differ, we first have to understand what the distribution of real people's birthdays looks like.
Real birthdays are not distributed equally throughout the year – if they were, we'd see each day representing 0.27% of the total. Instead, here are the ten most common birthdays in our "clear" dataset of non-synthetic identities (i.e. real people):
The first three dates are likely just data artifacts; people will occasionally enter dates like this if they don't wish to share their real information, and some systems may assign 1/1 as a default DOB if one has not been provided.
After that, the list is dominated by September birthdays. In the US, this is a known phenomenon, although nobody is certain why so many babies are born in September.
There are a variety of theories. It may be due to the fact that December – a romantic holiday season for many and the most popular month for engagements – falls nine months prior to September. Some scientists have also posited theories related to seasonal changes in hormone functions, sperm counts, and other fertility-related factors.
But whatever the reason, September is the most common birthday month, both in the US at large and in the dataset we analyzed here.
The least common birthdays among real people in our dataset also follow the patterns one might expect:
Unsurprisingly, February 29 – "leap day" – is the least common birthday, since it occurs just once every four years. After that come Christmas Day and Christmas Eve, with July 4th a little further down the list. These holidays are likely among the least common birthdays because medical interventions such as induced labor and Cesarean sections allow some mothers and doctors to schedule their births.
Beyond the birthday, though, real DOBs are distributed pretty evenly. For example, here's the distribution of birth years within our "clear" data set. It shows pretty much what we'd expect for the population we're sampling (primarily credit-active US residents):
Similarly, the distribution of days of the month is pretty even (the 31st is the least common day because five months have 30 or fewer days):
Now that we understand what the distribution of real birthdays looks like…
How do synthetic "birthdays" differ?
First and foremost: synthetic fraudsters apparently love "symmetrical" birthdays, perhaps because they're easier to remember. Here are the ten most common birthdays in our synthetic identity dataset. (For reference, if birthdays were distributed evenly throughout the year, each individual birthday would represent a 0.27% proportion of all birthdays).
As we can see, all but two of these dates are "symmetrical" birthdays such as 1-1 or 10-10. The two that aren't symmetrical are sequential birthdays: 1-2 and 11-12.
These birthdates occur much more often in synthetic identities than they do in real life. For example, while January 1 was the most popular birthday in both datasets, proportionally speaking there are still 4X more 1-1 birthdays among synthetic identities.
Overall, despite there being just 12 of them out of 365 days in the year, "symmetrical" birthdays make up 7.9% of all birthdays in the synthetic dataset, compared to just 3.6% of birthdays in the clear dataset.
The least common birthdays in our synthetic dataset also different significantly from the least common birthdays in the real world:
While leap day is still the least common birthday, the holidays are gone, and they've been replaced with a different pattern: all of these least-common birthdays fall very close to or precisely on the last day of the month.
We're not sure why synthetic fraudsters seem to dislike these dates. Our working theory is that they may tend to avoid dates at the end of the month so that they don't have to remember how many days a given month has.
The years synthetic fraudsters choose for their identities' DOBs also differ significantly from the distribution of real birth years:
As we can see, fraudsters are much more likely to pick more recent years, creating identities that are ostensibly in their twenties and thirties. They also particularly like the years 1990, 1999, and 2000; these years all appear 2-4X more in the synthetic dataset than in the clear dataset (proportionally speaking).
Finally, the distribution of days within the month that synthetic fraudsters choose is also unusual:
As we saw when we looked at the least common synthetic birthdays, fewer synthetic birthdays fall at the end of the month.
Conclusion
While the aim of most synthetic fraudsters is to create realistic-looking identities, the fact that they can choose elements of these identities such as their DOB leads to significant differences between the patterns we see in dates of birth in the synthetic and real populations. (We also see some interesting patterns in the names they choose, but we'll leave that analysis for another day.)
While it's fun to look at these patterns, synthetic fraud is a serious problem. Learn more about what synthetic fraud is, or book a demo to find out how SentiLink can help you stop losing money to synthetic and other forms of identity fraud.
Related Content
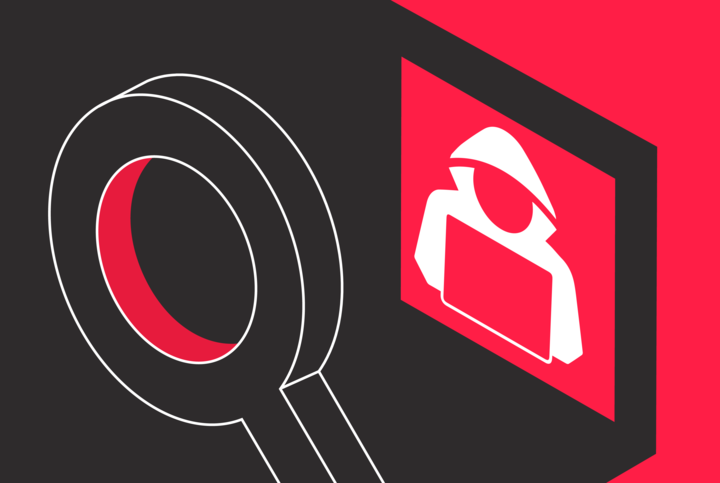
Blog article
March 11, 2025
Hands-On with the CPN Economy, Part 2: How CPNs are Sold and Marketed
Read article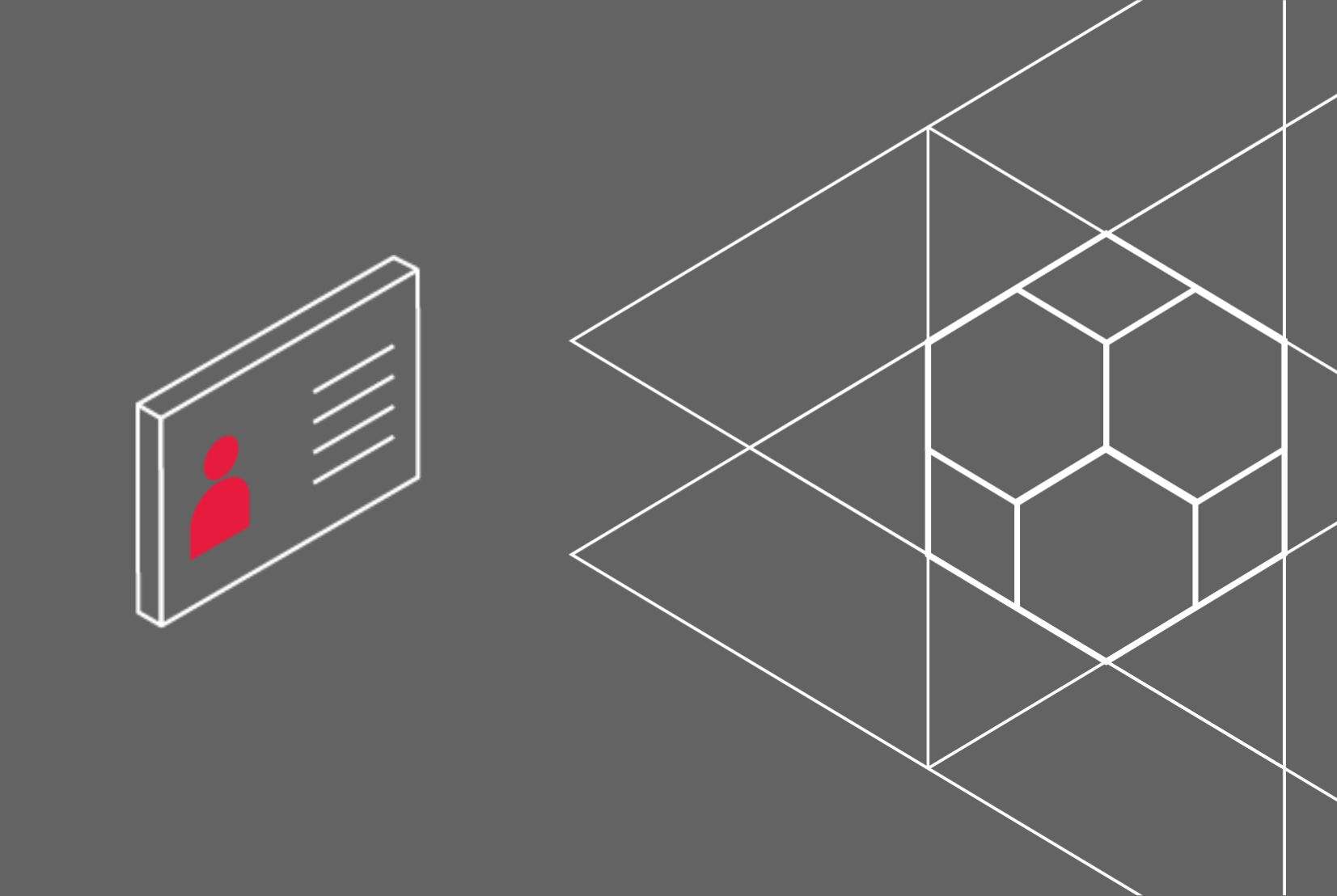
Blog article
March 10, 2025
Does Having a Common Surname Make You More Prone to Identity Theft?
Read article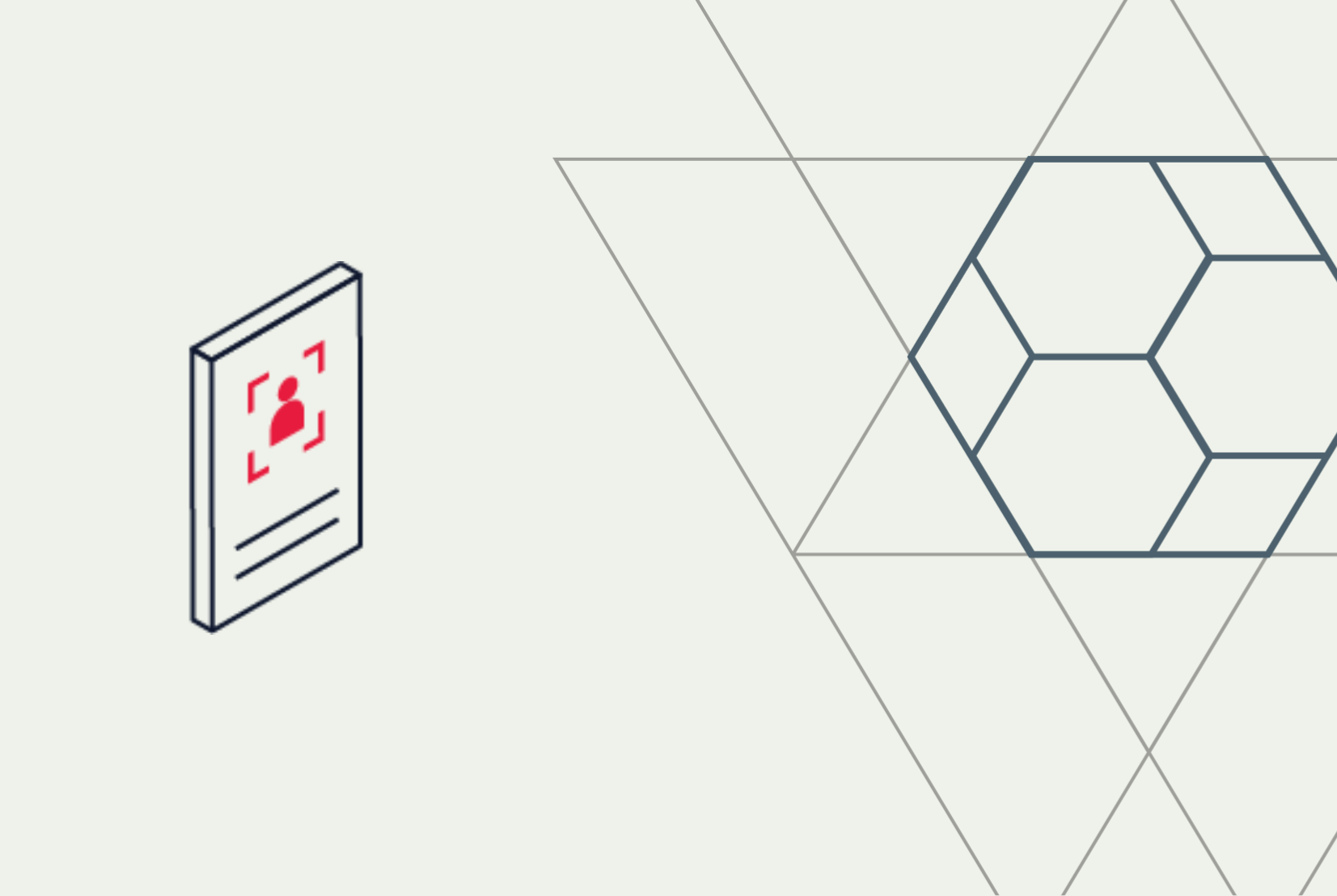
Blog article
March 5, 2025