Blog post
SentiLink’s Thoughts on GenAI Fraud
Naftali Harris
Published
June 3, 2024
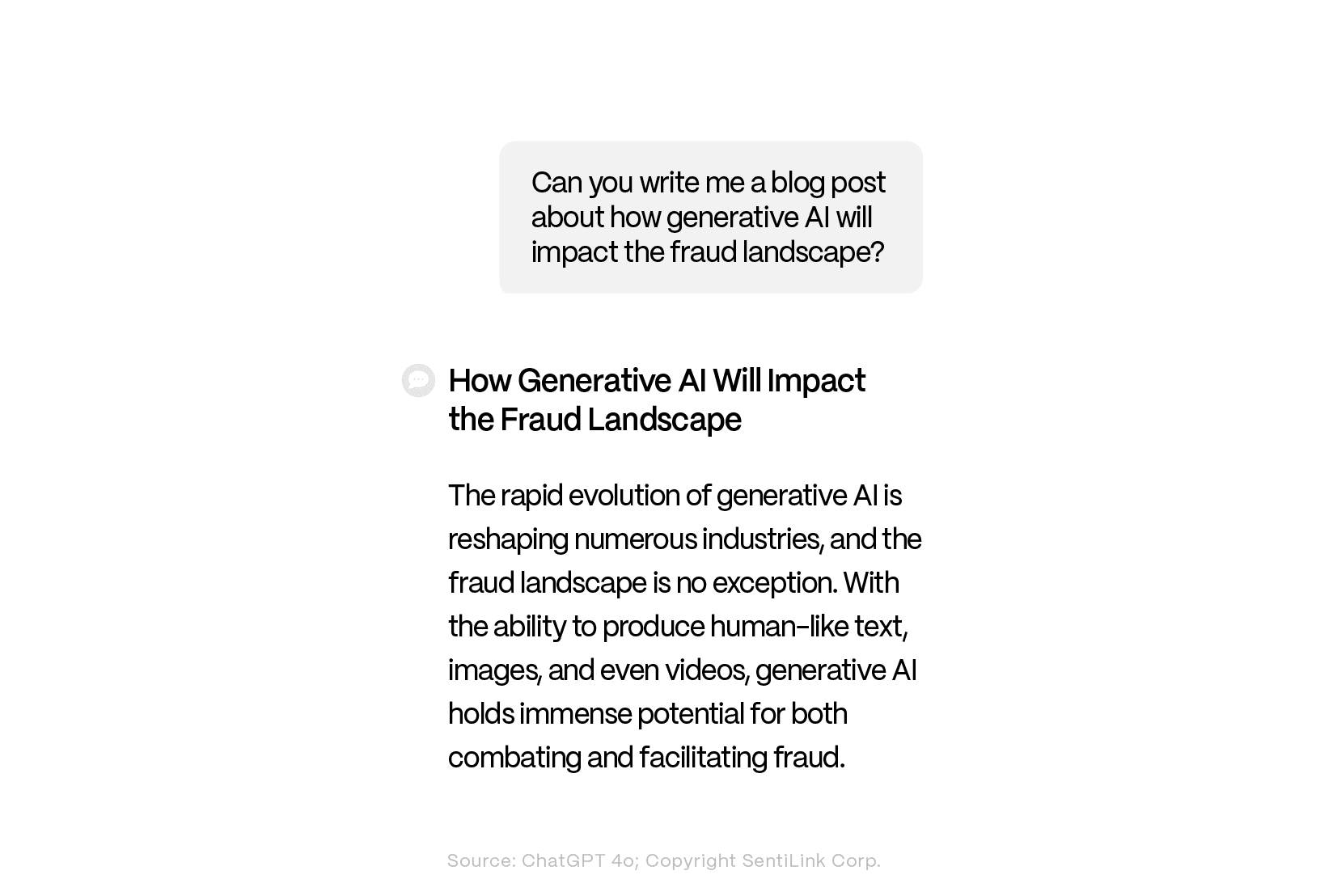
SentiLink is widely considered the market leader for synthetic fraud detection, so a question we get a lot is: “won’t GenAI lead to an explosion of synthetic fraud?”
The short answer to this is “no” (I’ll explain more in a bit). But we do think that GenAI will have a disruptive impact on fraud controls in other ways, and that the long-term equilibrium is non-obvious.
In this article I’ll walk through SentiLink’s thinking on the disruption GenAI will have on the fraud landscape, and also what we consider the most important open question on its long-term impact.
AI-generated synthetic identities?
But first, why won’t GenAI lead to an explosion of synthetic identities? After all, if GenAI is extremely good at creating realistic content, why wouldn’t this help fraudsters create synthetic identities faster or more convincingly?
The main reason is that synthetic identities are already very easy to create. To make one you just need a name, date of birth, and SSN. Names and DOBs are obviously easy to create without GenAI. You would think that the SSN would be the hard part, but it turns out that the structure of them is semi-public (before June 2011, the first five digits can tell you the state and year range when it was issued). In addition, the credit bureaus have no way of validating which SSNs were actually issued by the SSA, and so the act of repeatedly applying for credit with a fake SSN will actually create a credit record there. None of this requires or is materially accelerated by GenAI.
No, the challenge for fraudsters, and the bottleneck on creating synthetic identities, is establishing enough credit history for those identities to get decent credit scores and gain access to large amounts of credit. Fraudsters do this with techniques like authorized user tradeline purchases (“piggybacking”), which again isn't something generative AI can help with.
In addition, GenAI doesn’t really help bypass the treatment strategies for suspected synthetic fraud. Most directly, the best treatment strategy is eCBSV, where (with consumer consent) you can get a confirmation from the SSA about whether they actually have a record of the name/DOB/SSN combination. The most convincing AI-generated synthetic identity still can’t get the SSA to have a record that it doesn’t have.
Government-issued ID checks are another popular treatment strategy. But the majority of synthetic fraud is what we call “first party synthetic fraud”, in which a consumer with bad credit or fraudulent aims uses their true name and DOB but a fake SSN (or “CPN”) in order to get a new, clean credit report. These consumers can use their own, true, government-issued IDs (which have their names and DOBs on them but not their SSNs); they don’t need GenAI to create fake ones. (As an aside, this is why there’s still a lot of synthetic fraud in auto lending, despite the fact that you generally need to show up in person and show your driver’s license to purchase a car).
Learn more about how synthetic fraud is defined and categorized.
What fraud controls are threatened by generative AI?
While we don't think GenAI helps synthetic fraudsters that much, some common as well as state-of-the-art fraud controls are definitely threatened by GenAI:
Liveness checks are sometimes used as a step-up form of identity verification when identity fraud is suspected. A typical solution might require the applicant to turn on their camera and perform an action on video. This type of control has been effective at combating identity theft because while an ID thief may have a variety of personal information about their victim, they did not have a way to generate a video of their victim performing a specific action in real time.
With generative AI, however, this is becoming possible. My colleague David Maimon, Head of Fraud Insights at SentiLink, has chronicled fraudsters' attempts to use deepfake videos to circumvent liveness checks. They're not all convincing, but as the technology improves and fraudsters' skills improve along with it, it will become increasingly difficult to tell the difference between video of a real person and an AI-generated deepfake created by an identity thief using their victim's likeness.
Voice printing is a method of confirming identity that works by comparing an user's voice to a prior record of how they sound. Again, the idea is that while an identity thief might have access to their victim's information and imagery, they could not effectively impersonate their voice.
GenAI, however, has made it possible to quite convincingly imitate a real person's voice. Given a recorded sample of the person's speech – which fraudsters could harvest from their victim's public social media videos – a variety of tools can now do text-to-speech using a very convincing synthesized version of the person's voice. GPT-4o is already able to speak with minimal latency, as well as vary its voice upon request.
Behavioral biometrics is a category that we believe will also be impacted. Behavioral biometrics measure the specific details of application usage patterns (mouse movement, keyboard presses, etc) to determine whether the user appears to be a human or a bot. A generative AI trained with human usage patterns could produce data indistinguishable from that of a real human, and some AI companies are already working on AI tools that humans can train to use their apps.
While you might think that it will be a while before someone would bother building an AI to imitate human use of a device, we suspect that attempts to get around behavioral CAPTCHA systems like Google reCAPTCHA will probably be the driving force behind this malicious research.
Document verification was not always a reliable form of identity verification even in the days before GenAI, as skilled image editors and forgers could produce convincing-looking fake documents. However, this forgery took time and skill. GenAI tools are making it faster and easier for anyone to generate photorealistic images, giving more fraudsters access to this technique and allowing them to use it at greater scale.
Scam and spam detection will become more challenging as GenAI gives fraudsters the ability to imitate the style and tone of any person or organization they're trying to impersonate. Most people can relatively easily detect scams and spam today, just because they look sloppy or suspicious. But with GenAI fraudsters will be able to create content that may be able to convince even the most suspicious, as we saw earlier this year when fraudsters scammed a multinational corporation out of $25 million by tricking its finance team using a deepfake video call impersonating the company's CFO.
The biggest open question
Given these challenges, some people have suggested “using AI to detect AI”, i.e., building AI/ML models to figure out if content is machine-generated or not. To be sure, with the current state of GenAI, there are situations where this works. If you can be confident that some content is AI generated, it’s pretty much a slam dunk that there’s some kind of fraud, in the same way that finding “photoshop” in image EXIF metadata is a slam dunk that an image is edited.
But there’s a big open question which isn’t getting enough attention: as GenAI technology continues to improve, will it be possible in the future to distinguish generated content from the real thing?
We don’t think there’s an obvious answer to that.
Take a close look at the following images, for instance. Can you tell which of these two is AI-generated, and which is a real picture?
As you perhaps can see from this example, GenAI is already at the point where the average person often can't tell the difference between what is real and what is fake. That’s not to say that machines can’t do it, but we don’t think we should necessarily assume that this will always be the case as the tech continues to improve.
Fast forward a few years, and we could live in three possible worlds: one where it’s possible to consistently detect AI-generated content, one where there’s a cat and mouse game between GenAI and detection models, or one where AI-generated content is indistinguishable from human-generated content.
In fact, for GAN-generated content, there’s a natural argument that machines won’t be able to distinguish fake from real. GANs (“generative adversarial networks”) are an approach to generating realistic content where you train two “adversarial” models at the same time: one is a model that creates the content (the “generator”), and another is a model that tries to distinguish generated content from the real thing (the “discriminator”). You train the models until the discriminator isn’t able to tell the types of content apart. Avoiding detection is literally part of the training!
(To be fair, getting GANs to generate arbitrary content is an active area of research, so this approach may or may not work for the fraudsters).
As for those two images above… trick question! They're both AI-generated by https://thispersonnotexist.org/, which uses a GAN-based model.
GenAI proofing
If it might not be possible to detect AI-generated content in the future, and AI may be able to subvert a number of the industry’s fraud controls, what should we do about it? We have two suggestions:
First, identity verification solutions should increasingly rely on historical information. While GenAI may be able to create convincing fake videos of a person to defeat liveness checks, for example, it cannot create fake history such as historical ties to an address, phone number, or email. Even simple techniques like having the applicant verify control over an email address that has been tied to them for a long time can be a relatively strong and AI-proof approach. Put simply, GenAI can fake the present but it can’t fake the past.
Second, companies should increasingly lean into authoritative data sources such as eCBSV and AAMVA. As we discussed earlier, the most convincing AI-generated identity still can’t get the SSA to recognize an SSN it didn’t issue. Similarly, the most advanced GenAI can’t get a state DMV to recognize a fake driver’s license that it didn’t issue. GenAI may be able to trick humans (and maybe other machines), but it can’t create records that don’t exist.
Related Content
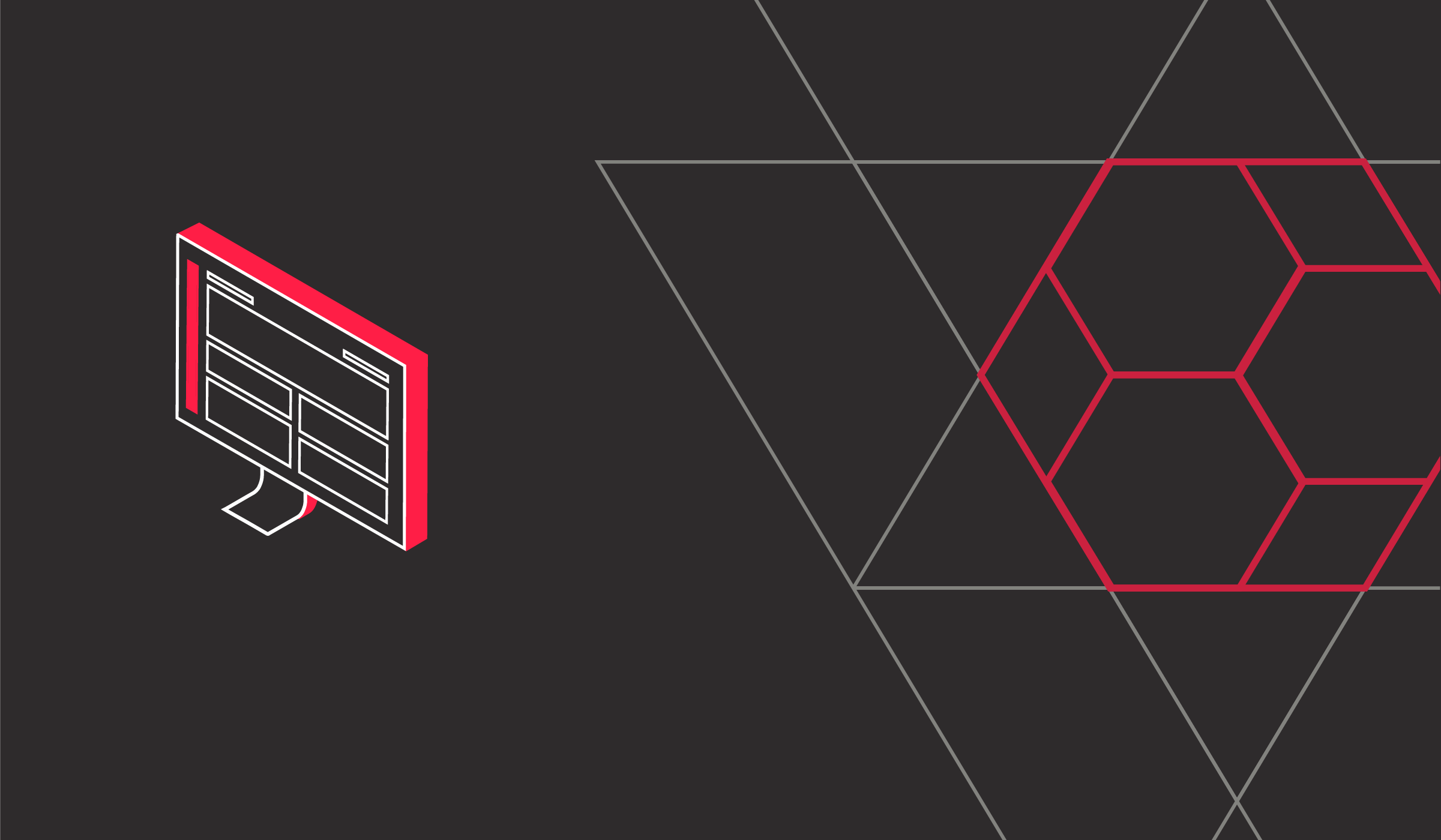
Blog article
January 13, 2025
2024 in Review: Enhancing Fraud Fighting with the SentiLink Dashboard
Read article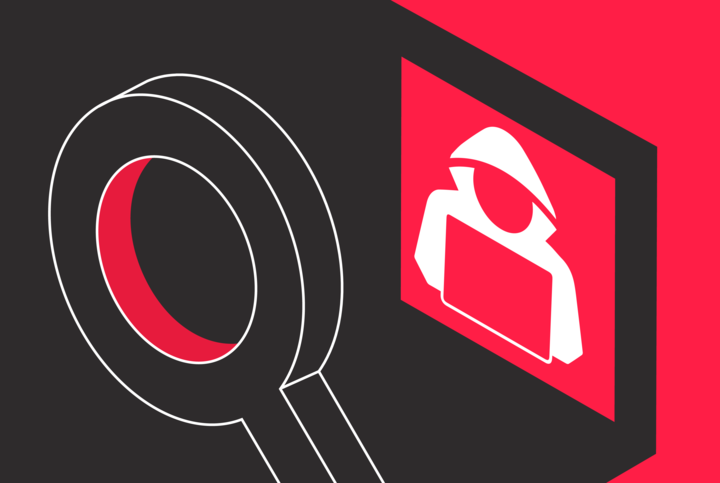
Blog article
December 19, 2024
Hands-On with the CPN Economy, Part 1
Read article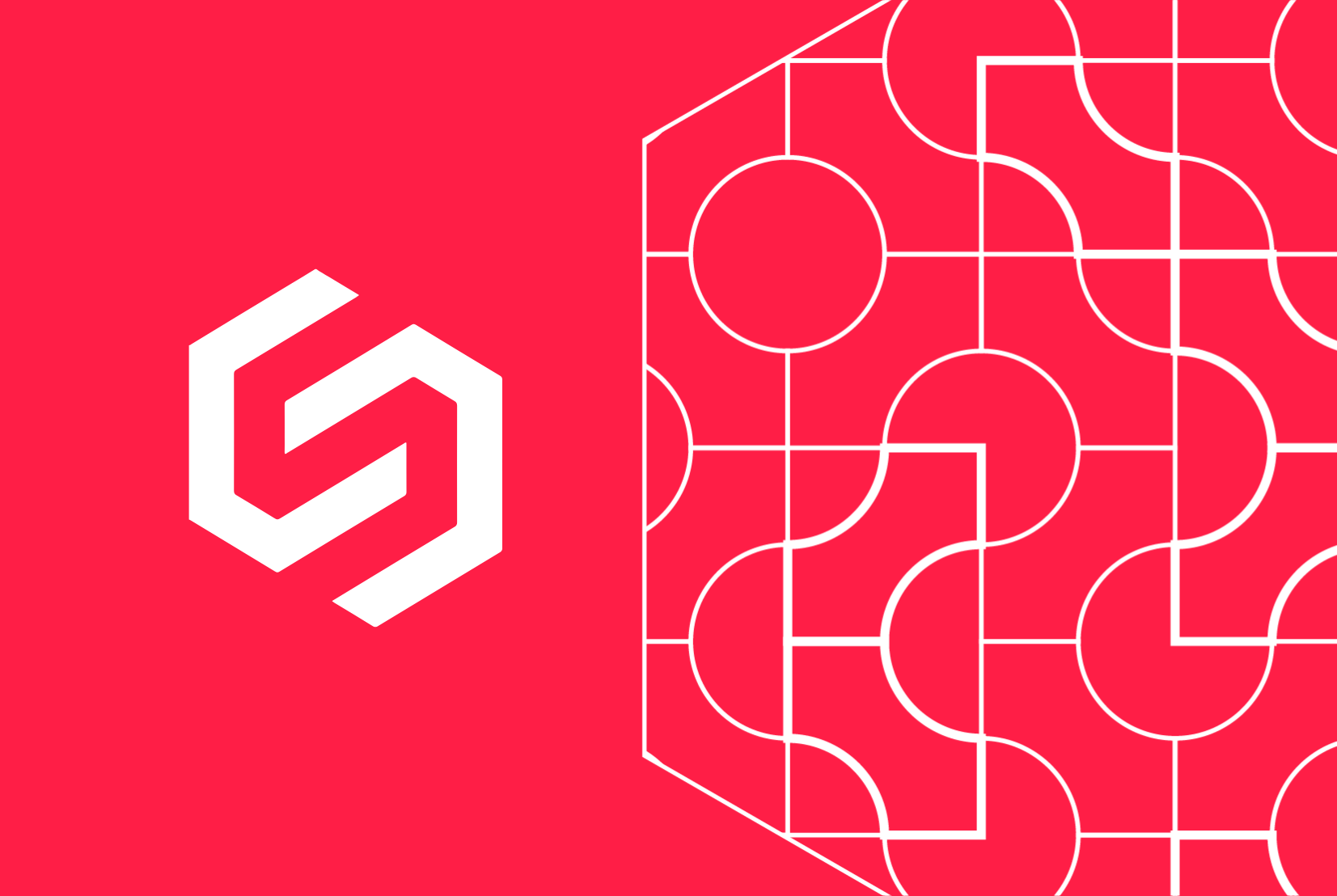
Blog article
December 11, 2024