Blog post
Same Name Fraud and the Case of the "Bad Justin"
Karl Lubenow - Fraud Intelligence Team
Published
October 30, 2023
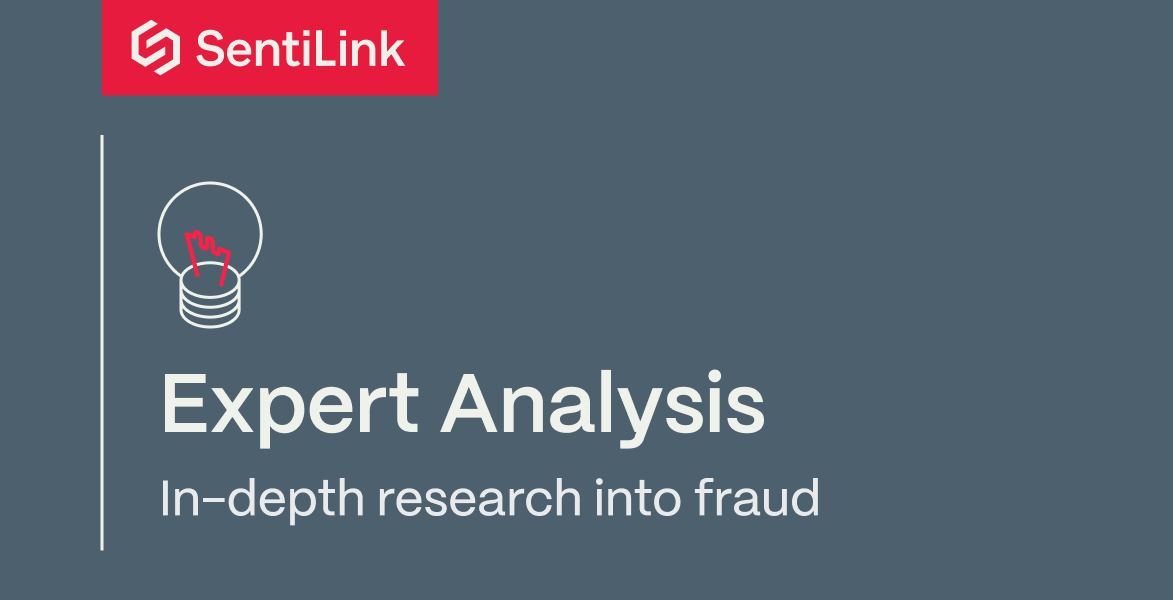
“How would you commit identity fraud?”
As a veteran fraud analyst, I get asked that question a lot. There is a common belief that those who work as fraud fighters would make excellent identity thieves ourselves. While it's true that I get a front-row seat to the latest and certainly most innovative fraud tactics, most like me in this field don't have the "criminal mastermind" gene built into our DNA.
In fact, most of the cases I review follow a few common patterns, such as purchased identities off the darkweb, successful phishing attempts, or a consumer being tricked into handing over a PIN sent to a phone or email to bypass 2FA. In other words, when you've reviewed thousands of cases as a Fraud Intelligence Analyst, it takes a lot to stand out. Sometimes though my colleagues and I see some really interesting types of fraud that are clever enough to make me think, well if I had to pick a type of fraud to try out for myself, this would be it. For me, it's "same name fraud."
“Same name fraud” is when a real person steals the identity of other people who share their same name. Unless that name is incredibly common, the people who are victims may only share a first and/or last name with the crook, with dissimilar DOBs and no similar address characteristics. None of this really matters, however: When it comes time to commit the fraud, the crook will just fill out an application (this can be in-person or online) with their own name, typically they will use their own actual DOB or the DOB of the victim, their actual address or the victim's address, the stolen or purchased SSN, but almost always will provide their own phone number and their own email address.
The reason this works is pretty simple: The usual checks performed on email and phone numbers at this point in an application flow will likely show a decent amount of history and connection to the name on the application. If 2FA is requested it's no problem since the fraudster controls the phone and/or email.
The Case of the Bad Justin
To help illustrate this, I'll walk through the elements of an actual case I reviewed that I call "The Case of the Bad Justin." This is based on a set of auto loan applications from earlier this year tied to a fraudster we believe to be based in the Chicagoland area. It involves one "Bad Justin" and four "Good Justins."
Bad Justin has a history of ties to 21 different SSNs he's used to commit synthetic fraud. He also has an extensive criminal record involving several dozen cases of identity theft, vehicle theft, forgery and various flavors of bank fraud.
|
I mentioned the concept of "seeding" a few times in this case to explain how Bad Justin was successful. What I mean by this is the process of a fraudster intentionally applying for loans with false information so that in subsequent applications months or years later, the information is perceived to be a match. This happens when a lender receiving an application attempts to pull the credit history based on the false PII provided by the fraudster. For our case, the similarities between Bad Justin's seeded credit reports and the established histories of the Good Justins can easily lead to a merging of credit identities at the bureaus. This is very common, especially with a parent and child who share similar names and overlapping address history. For Bad Justin, it makes his scheme much easier.
The whole point is to do just enough to get a lender to pull the credit report of someone else, who then becomes the victim of this type of fraud, along with the lender who will probably experience a quick default or bustout.
Stopping "Bad Justin" and same name fraud
While I've labeled quite a few cases as "same name fraud," there's no single magic bullet to catch it. At SentiLink, it takes a combination of our technologies to help us stop this type of fraud. For example, both our identity theft and synthetic fraud models were able to flag Bad Justin in every one of the applications that came in across our network. Having the benefit of that large consortium of lenders from a variety of industries and verticals made it possible for our linking analysis to see the behaviors Bad Justin was up to before the auto loan applications discussed in the case study were attempted. His exploits and my analysis are then used to train future updates to our models
Bad Justin did bad things, but SentiLink's ID theft and synthetic models were able to stop him. Good Justins can rest easier knowing that at least when SentiLink sees their identity used by Bad Justin, we are not fooled.
Related Content
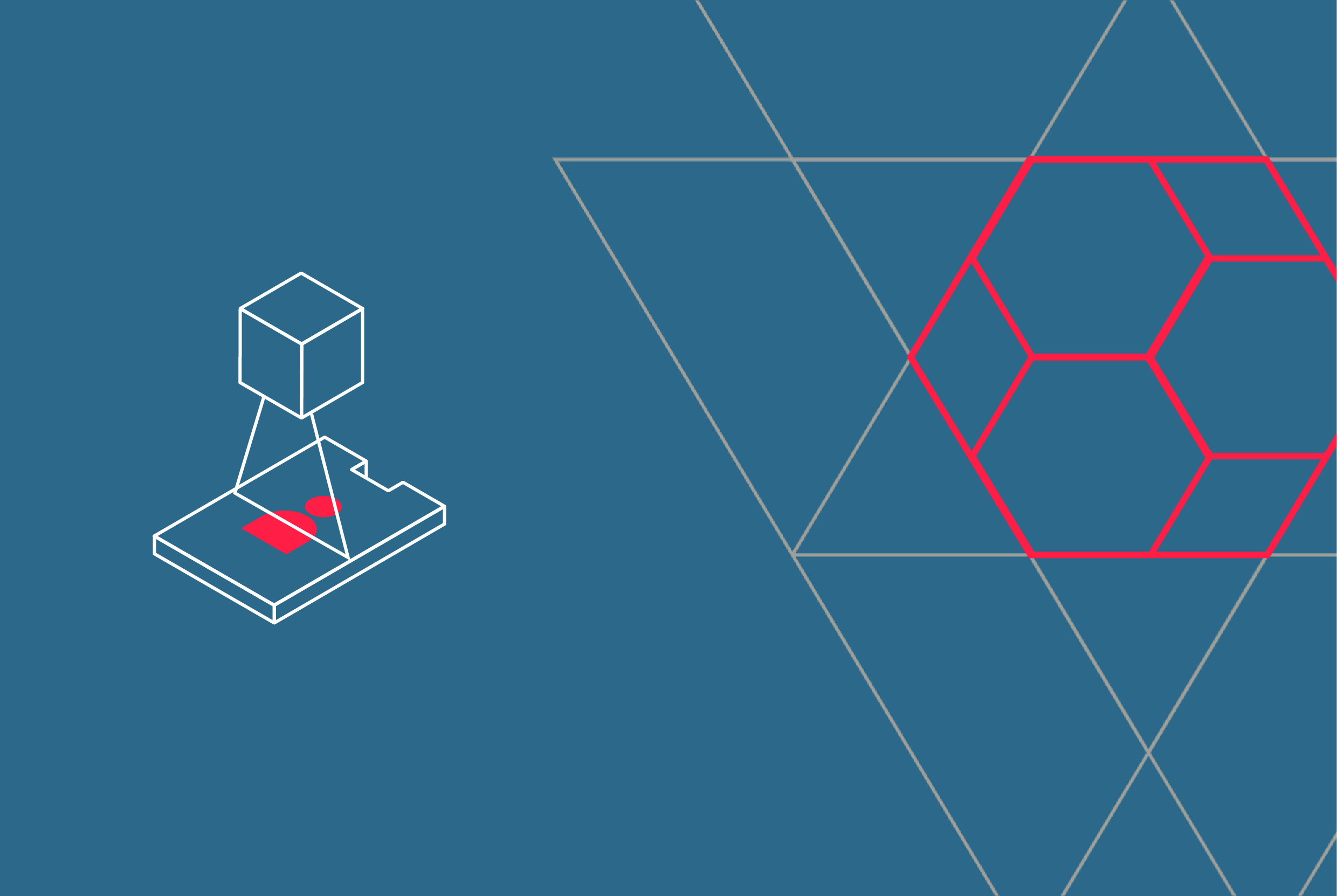
Blog article
April 24, 2025
What do M. Alvarez, J. Kim, and F. Mohamed have in common?
Read article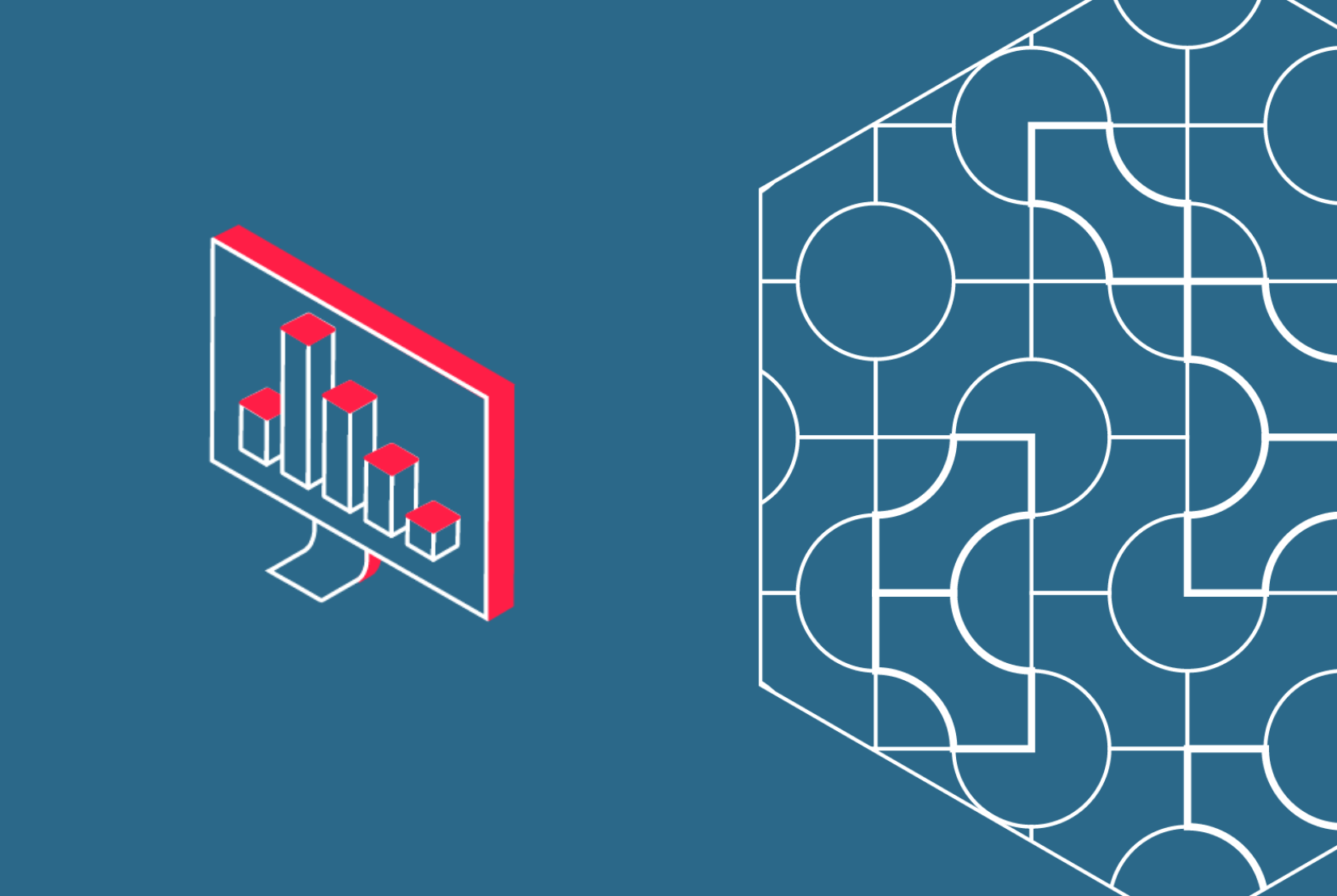
Blog article
April 18, 2025
What's Going on with SSA's "Death Master File"? (Part 2)
Read article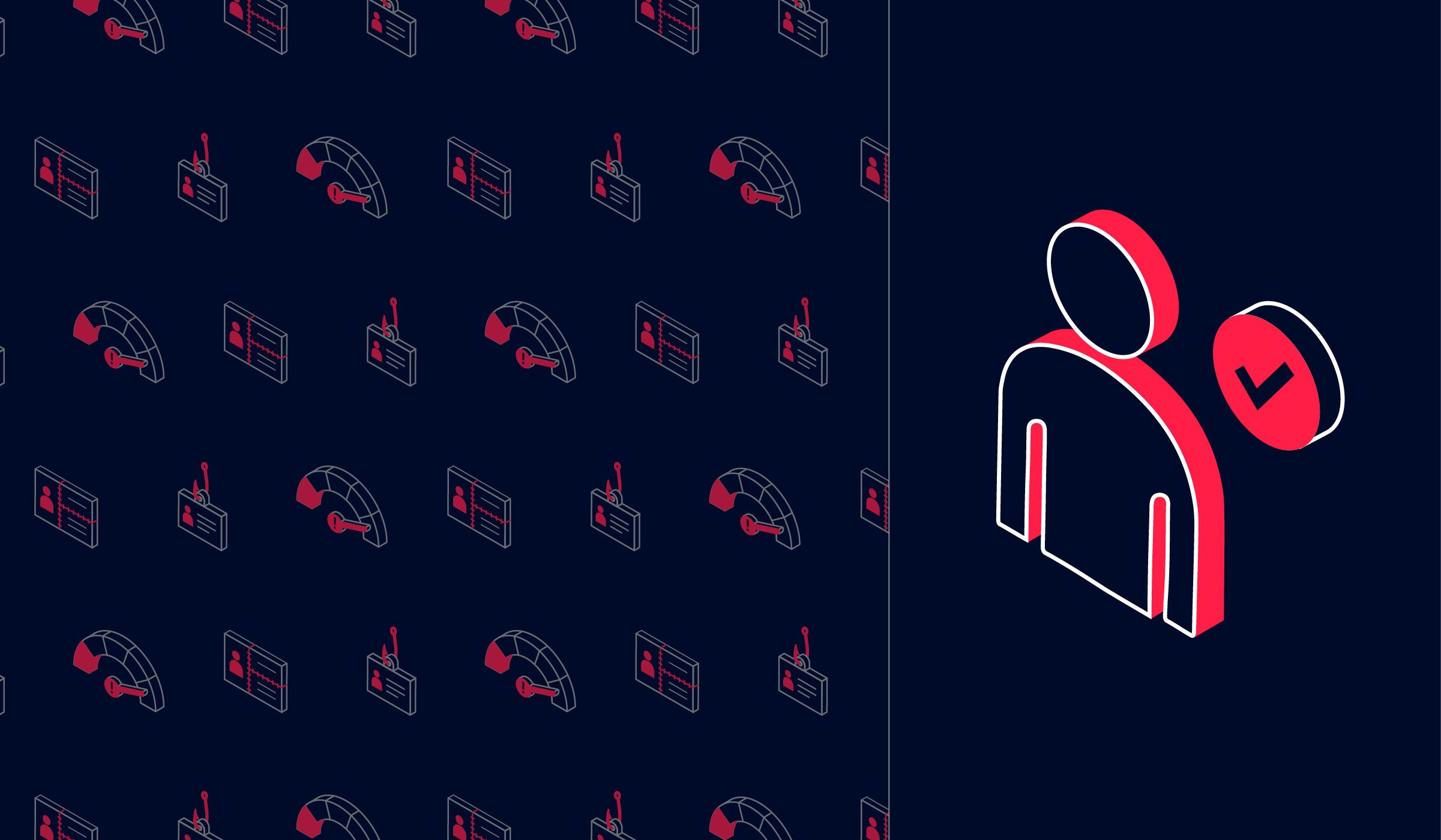
Blog article
April 17, 2025