Blog post
Reducing Complexity in Model Risk Management with Attributes
Michael Horrell, Staff Data Scientist
Published
February 29, 2024
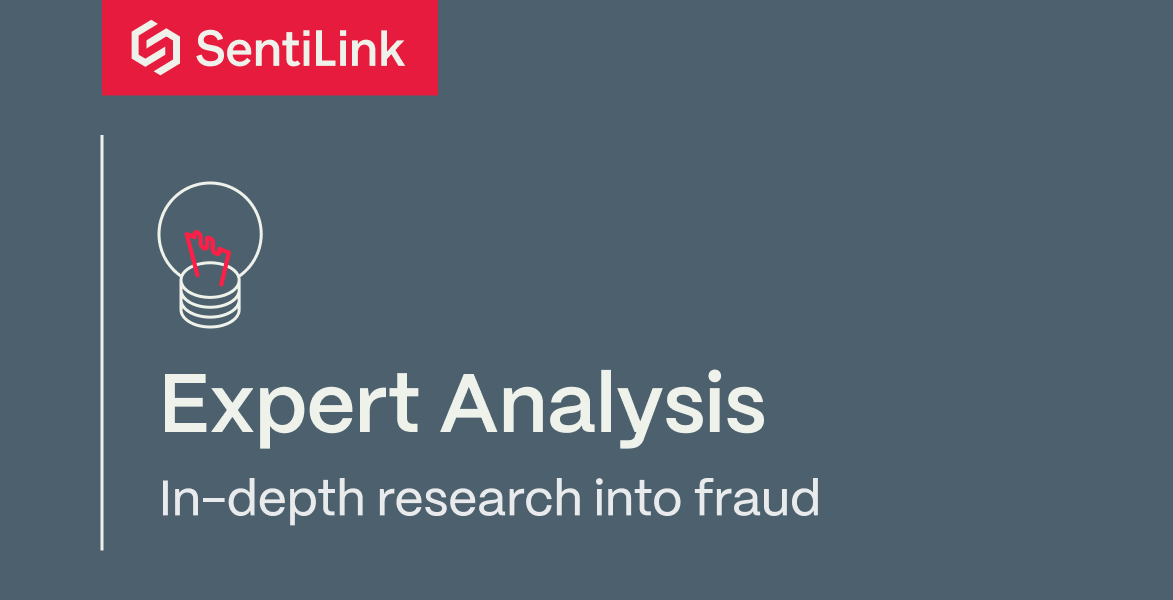
Model development and validation are central to the operations of financial institutions (FIs). For larger, complex banks, scrutiny on model functionality and customer impact is often a key component of regulatory examinations. With the focus and attention given to fair lending practices and BSA/AML compliance, FIs are challenged to make quick decisions via automation to ensure a great user experience, while meeting regulatory requirements of ensuring fair, functioning, and transparent models.
To help ensure in-house model development at financial institutions is consistent with safe and sound banking practices, federal banking regulators issued their Supervisory Guidance on Model Risk Management (SR Letter 11-7). Commonly, third-party data sources are used to improve model performance, though not all third-party data easily supports regulatory expectations. Attributes, a specific kind of third-party data product that can be used to directly augment a machine learning dataset, can both lead to empirically strong model performance and support adherence to these guidelines.
Integration complexity from third-party data
FIs building effective and fair in-house models for fraud prevention often buy products from third-party data vendors to improve the performance of their models. However, the use of third-party solutions can introduce complexity and uncertainty to model management, especially when a vendor may provide limited detail on the “secret sauce” of their algorithms or calculations. This makes the job of the model risk management team difficult when faced with requirements of validation and audit. Smaller institutions may face even greater difficulty due to limited resources to build and maintain models.
A strong model risk governance framework requires FIs to ensure that third-party vendor solutions are right for their needs. In SR Letter 11-7, regulators recognize the importance of data sources, including from third-parties, and place clear expectations on regulated entities to ensure such data used for model development is subject to strict review. For example (emphasis added):
The data and other information used to develop a model are of critical importance; there should be rigorous assessment of data quality and relevance, and appropriate documentation. Developers should be able to demonstrate that such data and information are suitable for the model and that they are consistent with the theory behind the approach and with the chosen methodology. If data proxies are used, they should be carefully identified, justified, and documented. If data and information are not representative of the bank's portfolio or other characteristics, or if assumptions are made to adjust the data and information, these factors should be properly tracked and analyzed so that users are aware of potential limitations. This is particularly important for external data and information (from a vendor or outside party), especially as they relate to new products, instruments, or activities.
Specifically, SR Letter 11-7 asserts three main components for an effective validation process:
A. Evaluation of conceptual soundness, including developmental evidence
B. Ongoing monitoring, including process verification and benchmarking
C. Outcomes analysis, including back-testing
Below we will refer to these components as SR 11-7 (A), (B) and (C).
Attributes and model governance
Generic third-party data sources will not necessarily align with the SR 11-7 requirements A-C. Attributes data products, which are intended to be easily integrated into any modeling process, will generally align with these requirements. Attributes products that best support model governance will have most or all of the following properties:
- Specific to the population of interest, ensuring relevance to the purpose of the model.
- Quantify important data characteristics on which an FI lacks full visibility, thus enabling the FI to more deeply assess aspects of its target data set.
- Report ground truth observations rather than ratings or predictions, providing consistency and reliability in their calculated values. In addition, each attribute should have directly interpretable definitions and values.
- Support “as-of” calculations, allowing for both real-time assessment of application details and point-in-time backtesting.
- Fast to query and easily concatenated to other data, empowering model builders with immediate value for development and intended use.
- Model-ready as Boolean, numeric or categorical values, and not overly generic; making it easy to include in a set of features and see immediate value.
Properties 1-3 support SR 11-7 (A), the conceptual soundness requirement. Attributes products providing clear visibility on the precise real-world measurements being provided ensure that the data points being used directly support the purpose of the model and provide a strong conceptual basis for use in modeling.
Properties 4-6 support SR 11-7 (B) and (C), the ongoing monitoring, outcomes analysis and back-testing requirements. Being able to see attributes values in an “as-of” way is critical for accurate backtesting and general research and development. Speed, always a welcome feature, also helps with monitoring as there is less friction to looking around more corners in any analysis. The model-readiness of any attribute also improves analysis capabilities by limiting further software development needed to, for example, turn a string into a numerical feature.
Conclusion
FIs grapple with an onerous task when assessing and validating in-house models, an issue compounded when solutions from external vendors are included in model development. Selecting a solution provider that will not only partner with the FI but also simplify the process of ensuring that the FI understands the solution is essential for maintaining compliant model risk and governance procedures, such as those stated in SR Letter 11-7.
At SentiLink, we recommend FIs consider Model Risk Governance complexities when selecting third-party vendor solutions for their data needs and model development. Our Facets are attributes infused with fraud, risk and identity intelligence for both supporting model risk management and governance as well as capturing fraud nuances in populations specific to an FIs business and products.
To learn more about SentiLink Facets and how we support model risk management and model governance, please reach out to us.