Blog post
When Failing an eCBSV Verification Isn't Indicative of Risk
Patrick Eckerle, Head of Analytics - SentiLink
Published
June 9, 2023
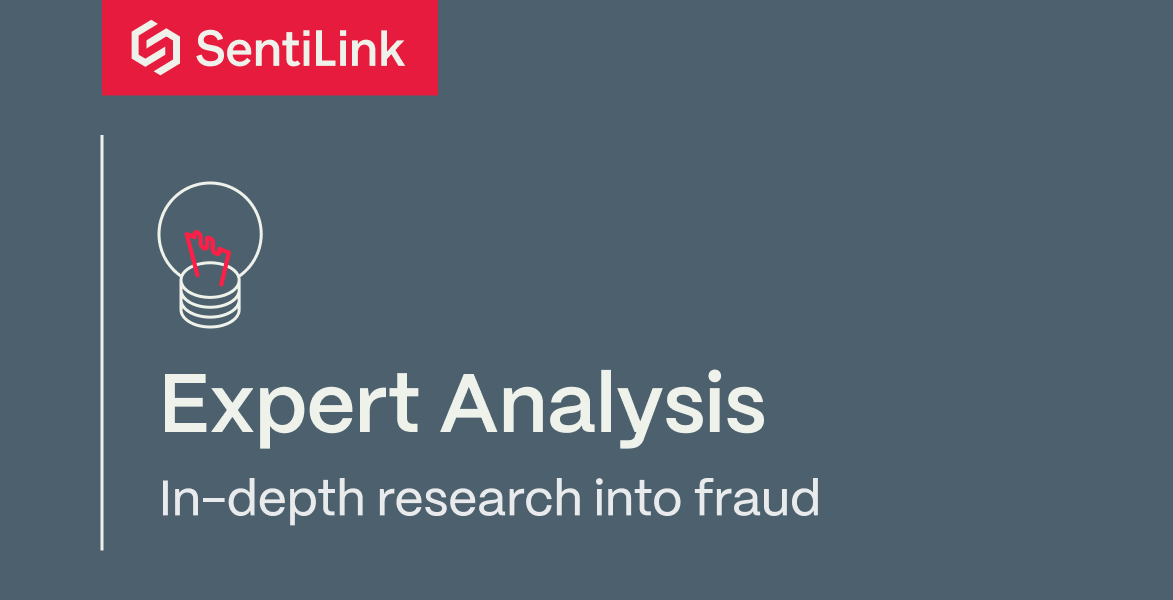
An Analysis of eCBSV Mismatches
In 2018, the SSA began development of a real-time, API-based system to allow the financial industry to verify a name, date of birth (DOB) and SSN combination electronically. Now operational, it is known as the Electronic Consent Based SSN Verification service, or eCBSV. Since going live, more than 137M verification requests have been made by all users of the system. SentiLink was the first provider in history to submit a name/DOB/SSN combination for verification with this new service. For a deeper dive into the system, please read our White Paper.
The primary goal of using eCBSV verification is to eliminate the risk of synthetic fraud by relying on the SSA to confirm that the provided information all belongs to a real person. Therefore, a No response from eCBSV would ideally represent a fraudulent or otherwise suspicious application. This is not always the case, though. A notable shortcoming of eCBSV is the relatively high mismatch rate--i.e., when some aspect of the name/DOB/SSN combination submitted by a financial institution does not correspond to a record on file with SSA, and the system returns a "No Match."
As of this writing the mismatch rate is 7.7%, according to SSA. While this is trending slightly down from historical average monthly mismatch rates, it remains notably high for most financial institutions. We've looked closely at many of these mismatches, and when we are able to isolate a No response from the SSA that we can confirm is not fraud, oftentimes we can show that a mismatch on its own does not represent a credit risk, either.
What Fraud Does eCBSV Find?
We believe 41% of eCBSV failures are cases of fraud. We consider most of these failures as synthetic fraud. This synthetic fraud is largely made up by either first party synthetics, where an applicant uses much of their own identity elements in the application – specifically their own name & DOB – but uses a falsified SSN. This is often done to cover up a prior bankruptcy or bad credit.
The other large portion of fraud that fails eCBSV we classify as third party synthetic. In the case of the third party synthetic, the applicant's identity is constructed of an entirely fabricated name, SSN/ITIN and DOB combination that the SSA would not recognize as belonging to a real person.
Beyond Synthetics
We believe first party and third party synthetics make up the majority of the fraud that eCBSV helps to identify. However, there are several other types of fraudulent behavior, with varying degrees of malicious intent, where an eCBSV mismatch may actually help a financial institution rule out other forms of fraud. Examples of this behavior within SentiLink's proprietary fraud taxonomy include:
- “Bad Juveniles”: An applicant is misrepresenting their birthday to appear over 18. We tend to not believe this is a large fraud risk for FIs, but a strict application of a "no" response from eCBSV could help to eliminate these users from the platform.
- Mixed PII between spouses: Combining PII elements between the applicant and their spouse. This is often observed with in-store financing/credit card applications where potentially one spouse did not know the other’s SSN but had already entered their own information. This is usually not malicious, but most lenders and credit products would not see these customers as underwritable since they would also fail the credit bureaus' identity check prior to a credit pull being available. Financial institutions looking to ensure all of the customers information is completely accurate could use eCBSV to know that the inputted information does not match for one identity.
Although these may not be synthetic fraud, FIs may in fact prefer to decline applicants who exhibit these behaviors. In those scenarios, actioning on a negative eCBSV response, usually through an enhanced authentication step, would be a value add.
What we see in non-fradulent mismatches
When we first began looking at the implementation of eCBSV, we hypothesized that an application that failed eCBSV but scored low on our synthetic fraud model did not represent any kind of fraud. We have recently been able to confirm this hypothesis by using a random sample of credit report tradelines combined with a statistically significant set of eCSBV failures that we are able to identify as non-fraudulent.
To complete this analysis, we used the SentiLink Abuse Score to determine the synthetic classification for each identity for which we had eCBSV results for. Developed based on first hand experience finding fraud in financial services, the SentiLink Abuse Score works to determine the probability that an applicant is a synthetic identity. We use machine learning to help scale the knowledge from our manually reviewed applications, allowing us to infer fraud based on what we have detected in the past. Our machine learning models work by comparing the information provided to the credit bureaus and other consortia data elements associated with that person to estimate the likelihood that an applicant is real. While our Abuse score typically scores the risk on a scale of 0-1000, we have grouped the score into 3 buckets - clear, risky and synthetic - to approximate the overall risk that an identity is a synthetic identity. For these purposes, we believe a clear app has little to no chance of being a synthetic identity.
As expected, eCBSV failure alone is not enough to differentiate between a risky customer and a safe customer. When looking at the tradelines for loans and credit cards, we actually find that eCBSV failures perform marginally better than eCBSV passes once the risk of synthetic fraud has been eliminated based on the SentiLink abuse score. This is demonstrated in the following chart which shows the charge off rates by month for the first twelve months on book for these tradelines. Overall, eCBSV failures that we are able to identify as non fraudulent actually have credit performance slightly better than the corresponding non fraudulent eCBSV passes. This could be related to the biases of the types of people likely to fail eCBSV without being fraudulent. The clear explanation of whether these failures are indeed less risky than a corresponding non fraudulent pass is germane but out of scope; what the analysis instead makes clear is that a eCBSV failure on its own does represent credit risk once the fraud concerns have been ameliorated.
How exact matching can go wrong
Any model or flag meant to propose a course of action based on a result is at risk of having false positives or false negatives. eCBSV is no different. It is easy to consider some cases where someone might “mismatch” their identity elements against what the SSA has on file that would generate a failure when the applicant is indeed real. Typos and nicknames are two obvious examples. Ideally a lender would not want to decline "William Clinton" for a loan if he input his name as "Bill Clinton" simply because his birth certificate has "William" on it. We can, in fact, confirm that these types of pedantic typos do not generate a eCBSV failure as 99% of inputted identities with an exact match on DOB, SSN & Last name do not generate an eCBSV failure. That is not to say that no typos can generate an eCBSV failure. Indeed, we believe that 46% of eCBSV failures are cases where the inputted data is a close match to the known identity in the SSA's database that we believe are not fraudulent.
The issues we do see with the exact matching utilized by the SSA include:
- Maiden name mismatches
- Typos over a certain level of difference
On the first point, SentiLink could not find any studies related to when and if recently married or divorced women actually change their name with the SSA, so we performed an online survey of 244 responses from women to a set of six questions related to their name change activity with the SSA when they were married and also when divorced, if they did divorce.
While not wholly scientific in nature, the results mirrored other similar surveys. We found that 90% of women who marry and change their name contact the SSA and formalize the name change. 60% of those women who changed their name with the SSA make the change in the first 30 days of their wedding and another 28% change their name within the year. Less than 2% of women who change their name say they have no plans to formalize the name change with the SSA.
Among women who divorce and change their name, 42% recalled taking at least 3 months to change their name with the SSA, with 29% that either took at least a year or have not updated their name yet.
Naming Conventions: A surname, family name, or last name is the portion in some cultures of a personal name that indicates a person’s family, community or tribe. Depending on the culture, all members of a family unit generally have identical surnames with some variations.
In the English-speaking world, a surname is commonly referred to as a "last name" because it is usually placed at the end of a person’s full name, after any given names. American naming conventions are fairly simple and well-understood, and even variability to a person’s name related to nicknames and changes are a part of our everyday matching technologies.
In other parts of the world naming conventions may not be as simple, and therefore international names can create problems for US-centric identity matching systems. Unique cultural differences in the ordering of names, name changes due to marriage, alternate date notations, broader use of hyphenation and apostrophes, and multiple surnames can wreak havoc as records are formatted and reformatted while moving through a progression of systems — from consumer to financial institution to solution provider to SSA/eCBSV and back.
Conclusion
Receiving a binary Yes or No from eCBSV on its own is missing important context. For No responses in particular, the lack of any additional insight as to why the mismatch occurred makes it difficult for a financial institution to confidently determine whether an application is fraud or not. Our analysis shows the need for financial institutions to utilize a score like the SentiLink Abuse Score to accurately distinguish between fraud attempts and non-fraud mismatches due to typos, ITINs and other non-malicious factors.
Related Content
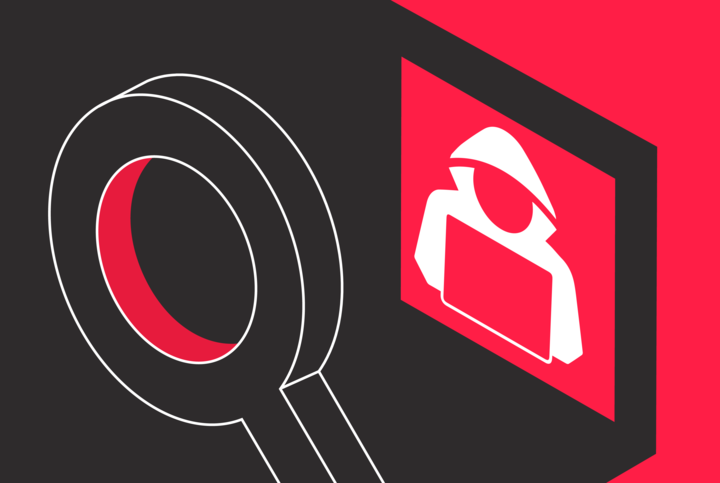
Blog article
January 29, 2025
We analyzed $77M worth of stolen bank accounts. Here's what we found.
Read article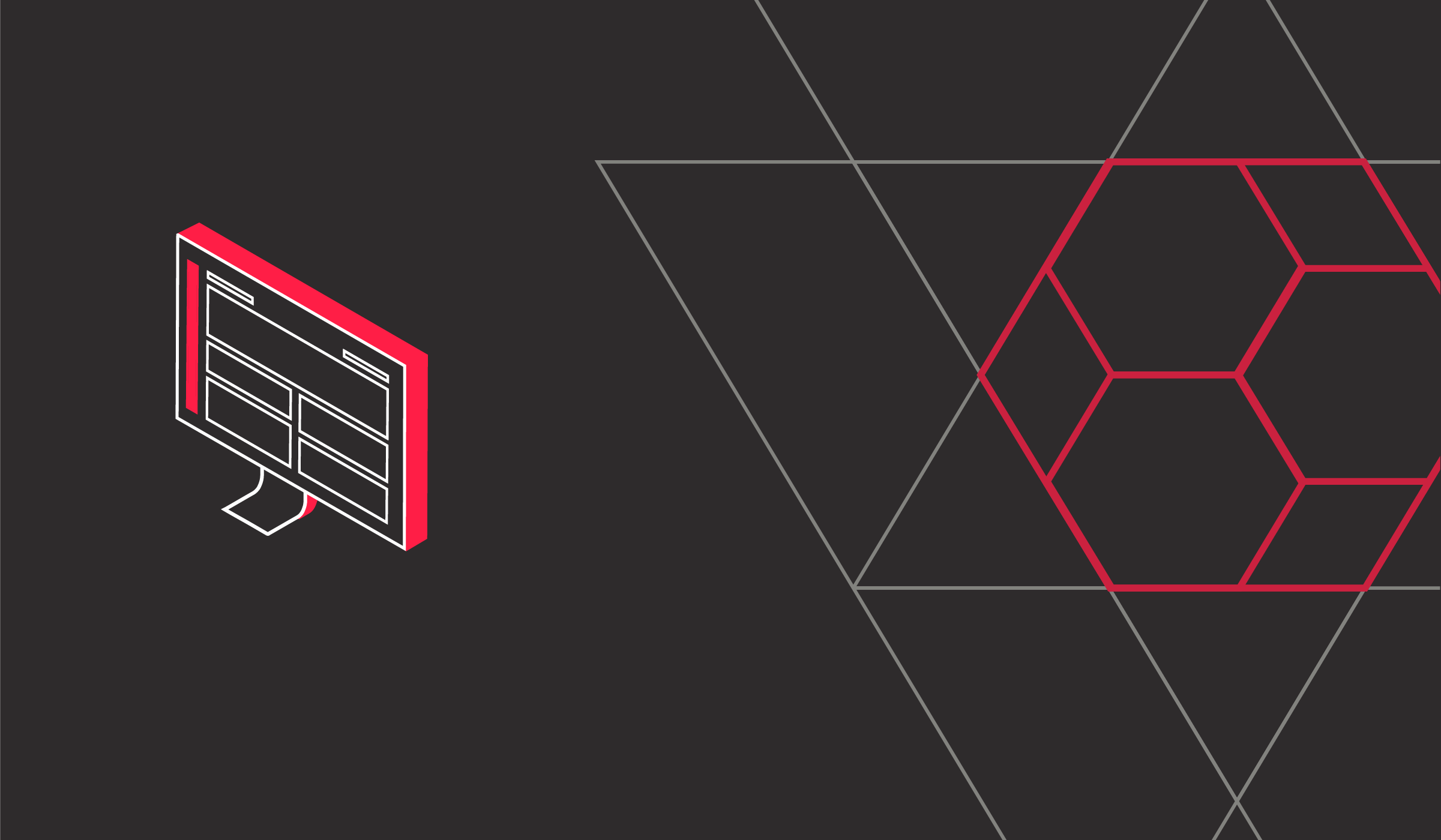
Blog article
January 13, 2025
2024 in Review: Enhancing Fraud Fighting with the SentiLink Dashboard
Read article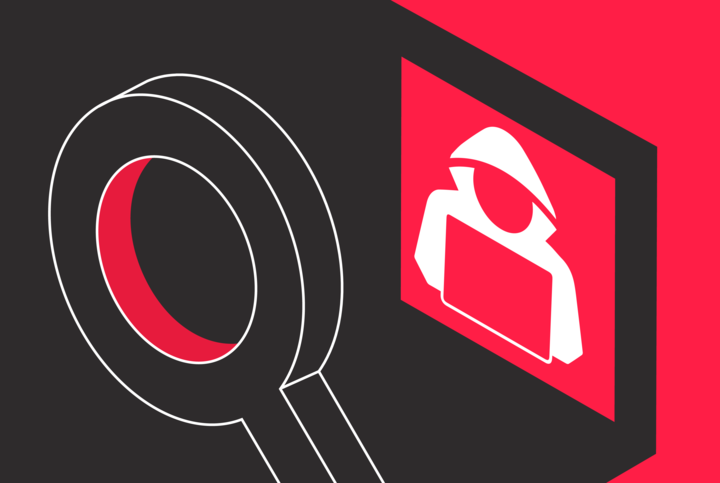
Blog article
December 19, 2024